You can also be interested in these:
- NVIDIA GeForce GT 720 review
- NVIDIA GeForce GTX 1050 Ti Max-Q review
- NVIDIA Quadro FX 3800 review
- Nvidia GeForce RTX 4090 full review
Ada Lovelace was a British mathematician and writer, known for her work in the field of computing. She is known as the first programmer in history, thanks to her collaboration in the development of the first algorithm intended to be executed by Charles Babbage’s Analytical Machine. Ada Lovelace wrote about the Analytical Machine and its potential for applications beyond purely mathematical ones, which makes her an important figure in the history of technology and computing.
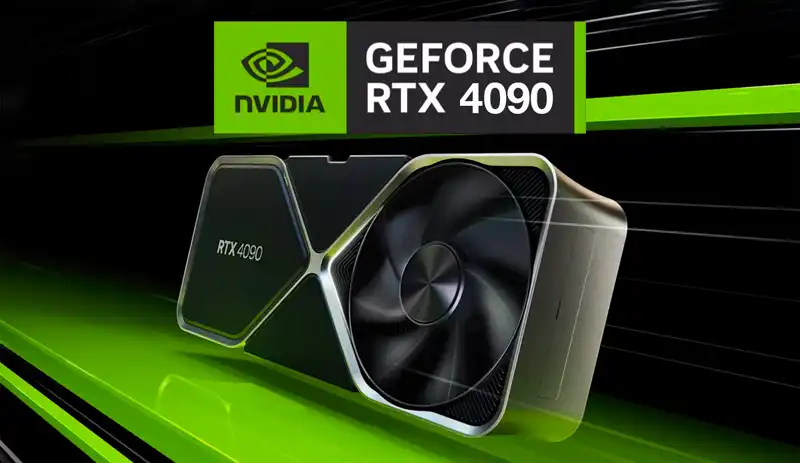
Mid-20th century, decades after her death, researchers found her work and a series of contributions that turned out to be very important for the progress of computing and programming. The historical significance of this visionary woman is unquestionable, which is why NVIDIA decided to pay homage to her and name its GeForce RTX 40 GPU family after her. All the features included in this new generation of GPUs is what Nvidia calls the Ada Lovelace Architecture. Now, let’s see what these features are all about.
Reference on the Nvidia RTX 40 Series
The first Nvidia RTX 4080 and RTX 4090 graphics processing units that will arrive in the market will have two versions. One of them with 16 GB of GDDR6X VRAM, and the other 12 GB. In the same line, they will also have a different memory bus. What is really relevant is that this new series will be produced on the 4 nanometer lithography node.
NVIDIA qualifies the implementation of the Ada Lovelace technology as a huge advance in terms of performance and efficiency. Let’s see if this statement honors the actual performance of the product.
Regarding integration technology, NVIDIA has opted for 4 nm TSMC instead of 8 nm Samsung used in the manufacture of graphics processors in the GeForce RTX 30 series. Furthermore, the new GPUs include new RT and Tensor cores, as well as a larger number of CUDA cores, along with higher clock frequencies and advanced image processing technologies, like DLSS 3.
More Processing Cores
The CUDA cores are responsible for performing complex calculations that a GPU needs to resolve, such as global illumination, shading, anti-aliasing, and physics. These algorithms benefit from an architecture that prioritizes massive parallelism, so each new generation of NVIDIA GPU includes more CUDA cores.
RT cores are the units responsible for taking on a large portion of the computation work required for ray tracing image rendering, freeing up other parts of the GPU that are less efficient in this task. To a great extent, they are responsible for the GeForce RTX 20, 30, and 40 series graphics cards being able to offer real-time ray tracing.
According to NVIDIA, their third-generation RT cores have double the performance of previous ones in processing triangle intersections in creating each frame. Additionally, these cores include two innovative engines, the Opacity Micromap (OMM) and the Displaced Micro-Mesh (DMM), which improve image creation efficiency.
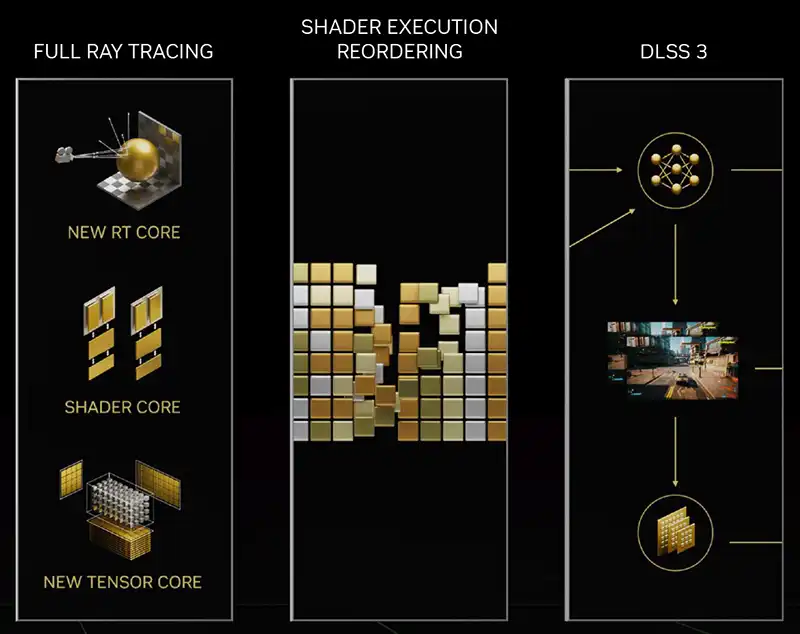
The OMM accelerates image creation through ray tracing in elements such as vegetation, barriers, and particles, easing the load on the GPU in these cases. On the other hand, the DMM processes scenes with high geometric complexity to allow for real-time ray tracing.
The fourth generation of Tensor cores has also evolved. These cores specialized in matrix operations are now more efficient in executing deep learning algorithms and high-speed computation. These cores play a key role in the DLSS (Deep Learning Super Sampling) technology and stand out in image reconstruction using DLSS 3.
According to NVIDIA, the fourth generation of these Tensor cores is five times faster than the previous one, especially thanks to the use of the FP8 transformation engine and 8-bit floating-point calculation. This engine comes from the H100 Tensor Core GPU, designed by NVIDIA for artificial intelligence data centers.
Shader Execution Reordering (SER) and Ada Optical Flow Accelerator technologies
Real-time rendering with ray tracing requires a large number of computational resources, so each new generation of graphics cards cannot simply increase the number of functional units. Raw power is important, but it is not enough. It is also crucial to have strategies for addressing the rendering process in a more intelligent way.
The SER and Ada Optical Flow Accelerator technologies aim to increase GPU performance by handling rendering tasks in the most efficient way possible. This is the approach adopted by NVIDIA with its GeForce RTX 40 GPU. These technologies seek to improve GPU performance by efficiently addressing rendering tasks that require more computational effort.
The SER technology optimizes GPU resources by intelligently reorganizing shaders in real-time. These are the programs that perform the necessary calculations to determine the essential attributes of the frame such as lighting and color.
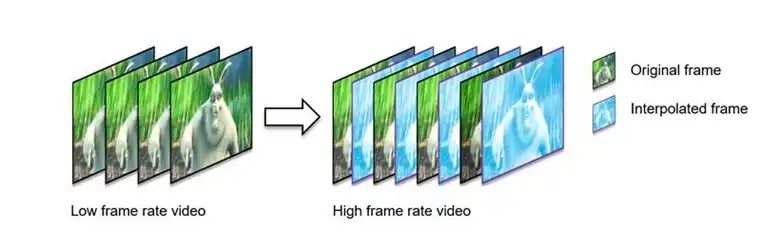
This technique is similar to CPU superscalar execution and, according to NVIDIA, allows the SER technology to multiply the ray tracing rendering performance by three and increase the frame rate by up to 25%. It has good potential.
Furthermore, the aim of the Ada Optical Flow Accelerator technology is to predict the movements of objects between two consecutive frames and provide this information to the convolutional neural network responsible for reconstructing the image with DLSS 3.
According to NVIDIA, this technique doubles the performance of the previous DLSS implementation and, at the same time, preserves image quality. It seems promising, but as it is such a new technology, users will have to verify it over time if it really meets the generated expectations.
The GeForce RTX 40 GPUs, according to NVIDIA’s promise, are more efficient than the RTX 30 due to being manufactured with the 4 nm integration technology from TSMC compared to the 8 nm lithography from Samsung used in the previous series.
Energy consumption and performance comparison
Compared in terms of energy consumption and performance, of the Turing, Ampere, and Ada Lovelace architectures, this latest implementation greatly surpasses the previous ones. However, we should not ignore the high electrical consumption of NVIDIA’s new graphics cards. The fact is that higher power means higher consumption.
According to NVIDIA, the GeForce RTX 4090 and 4080 reach a maximum temperature of 90 ºC under stress, but the average consumption of the RTX 4090 is around 450 watts, while the RTX 4080 uses around 320 watts. Additionally, NVIDIA suggests that the equipment using the RTX 4090 have a power supply of 850 watts or more, and that the RTX 4080 needs at least 750 watts.
The NVIDIA image reconstruction technique uses real-time analysis of game frames with deep learning algorithms. Its approach is similar to other graphics hardware manufacturers: the rendering resolution is lower than the final resolution delivered to the screen.
In this way, the load on the graphics processor is reduced, but in return, a process is required that scales the frames from the rendering resolution to the final resolution efficiently, otherwise, the effort that was avoided in the previous stage could appear in this stage of image generation.
Nvidia Deep Learning Super Sampling 3
NVIDIA’s artificial intelligence comes into play in this stage thanks to its GPU Tensor cores. The graphics engine first renders the images at a lower resolution, but then the DLSS technology increases them to the desired resolution through deep learning to recover the highest level of detail possible.
The process in DLSS 3 is more complex than in DLSS 2. In fact, this new NVIDIA image reconstruction technique uses the fourth-generation Tensor cores of the GeForce RTX 40 GPU to run a new algorithm called Optical Multi Frame Generation.
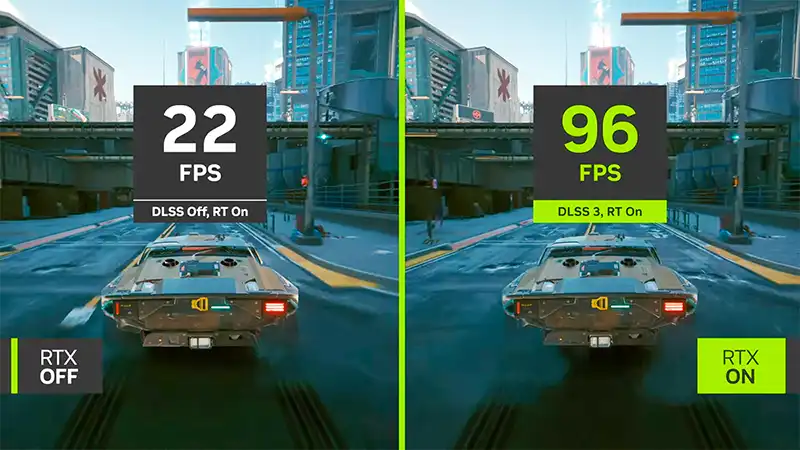
This algorithm analyzes two sequential images in real-time and determines the information in the vector that describes the movement of objects. Instead of focusing on the reconstruction of each frame separately, as DLSS 2 does, this strategy generates complete frames by analyzing the movement of objects in two sequential images in real-time.
According to NVIDIA, this reconstruction technique multiplies the frames per second rate offered by DLSS 2 by four and minimizes visual aberrations and abnormalities. In addition, the high-resolution frame processing and motion vectors are based on a convolutional neural network that analyzes all the information and generates an additional frame in real-time for each frame processed by the game engine.
Finally, DLSS 3 is compatible with Unity and Unreal Engine and will be available in over 35 games in the coming months. It can also be easily enabled in titles that already use DLSS 2 or Streamline.
More stories like this
- NVIDIA GeForce GT 720 review
- NVIDIA GeForce GTX 1050 Ti Max-Q review
- NVIDIA Quadro FX 3800 review
- Nvidia GeForce RTX 4090 full review
- Nvidia RTX 3090 vs RTX 3090 Ti: The not-so-obvious conclusion
- AMD Low Framerate Compensation (AMD LFC) explained